Data Cleansing vs. Data Scrubbing: What's the Difference?
Improve your understanding of data management with our comprehensive guide on the differences between data cleansing and data scrubbing. Discover how these practices enhance data quality, ensure compliance, and drive informed decision-making. Learn about the benefits, challenges, and best practices, as well as the role of data cleansing software in streamlining operations.
In the realm of data management, terms like data cleansing and data scrubbing are often used interchangeably, leading to confusion about their respective meanings and purposes. While both practices aim to improve data quality, they differ in focus, scope, and methodology. In this guide, we'll delve into the distinctions between data cleansing and data scrubbing, shedding light on their unique roles in maintaining data integrity and reliability.
Understanding Data Cleansing
Data cleansing, also known as data cleaning or data scrubbing, is the process of identifying and correcting errors, inconsistencies, and inaccuracies within datasets. The primary objective of data cleansing is to ensure that data is accurate, complete, and consistent, thereby enhancing its quality and reliability. This process involves various techniques and tools, such as deduplication, normalization, and validation, to identify and rectify data quality issues.
Understanding Data Scrubbing
Data scrubbing, on the other hand, focuses specifically on identifying and removing inaccurate, incomplete, or irrelevant data from datasets. Unlike data cleansing, which aims to correct errors and inconsistencies, data scrubbing involves the removal of data that does not meet predefined quality criteria. This process is often used to eliminate duplicate records, outdated information, and irrelevant data, ensuring that only high-quality data is retained for analysis and decision-making.
Key Differences Between Data Cleansing and Data Scrubbing
While data cleansing and data scrubbing share a common goal of improving data quality, they differ in several key aspects:
-
Focus and Scope: Data cleansing addresses a broader range of data quality issues, including errors, inconsistencies, and inaccuracies, whereas data scrubbing focuses specifically on removing irrelevant or redundant data.
-
Timing and Frequency: Data cleansing is typically performed as part of regular data maintenance processes, whereas data scrubbing may be performed as a one-time or periodic activity to clean up datasets before analysis or reporting.
-
Level of Automation: Data cleansing processes are often highly automated, leveraging algorithms and tools to identify and rectify data quality issues, while data scrubbing may involve more manual intervention to review and remove irrelevant data manually.
-
Application Areas: Data cleansing is commonly used across various industries and applications where data quality is critical, such as finance, healthcare, and retail, while data scrubbing is often employed in data warehousing, analytics, and reporting to ensure the accuracy and reliability of insights derived from data analysis.
Real-World Applications
In real-world scenarios, both data cleansing and data scrubbing play crucial roles in maintaining data integrity and reliability:
-
Data Cleansing: In a retail setting, data cleansing software may be used to identify and correct errors in customer records, such as misspellings or outdated contact information, ensuring accurate customer profiling and personalized marketing campaigns.
-
Data Scrubbing: In a healthcare environment, data scrubbing techniques may be applied to remove duplicate patient records from electronic health records (EHR) systems, reducing the risk of medication errors and improving patient safety.
Benefits of Data Cleansing and Data Scrubbing
Both data cleansing and data scrubbing offer significant benefits for organizations looking to improve data quality and reliability:
-
Improved Data Quality: By identifying and correcting errors and inconsistencies, data cleansing ensures that data is accurate, complete, and consistent, while data scrubbing removes irrelevant or redundant data, ensuring that only high-quality data is retained.
-
Enhanced Decision-Making Processes: High-quality data resulting from data cleansing and data scrubbing processes enables organizations to make more informed decisions, identify trends, and uncover insights with confidence.
-
Compliance with Regulatory Standards: Data cleansing and data scrubbing help organizations comply with regulatory requirements and industry standards by ensuring that data is accurate, reliable, and up-to-date, reducing the risk of non-compliance and potential penalties.
Data Cleansing Software
Data cleansing software plays a pivotal role in automating and streamlining the data cleansing process. These software solutions utilize advanced algorithms and techniques to detect and correct errors, inconsistencies, and duplicates within datasets, thereby improving data quality and reliability. By integrating data cleansing software into their workflows, organizations can ensure the accuracy and integrity of their data, enabling better decision-making and enhanced operational efficiency.
Challenges and Considerations
While data cleansing and data scrubbing offer significant benefits, organizations must navigate certain challenges:
-
Integration Complexity: Integrating data cleansing and data scrubbing processes with existing systems and workflows may pose technical challenges and require careful planning and coordination.
-
Data Privacy and Security Concerns: Organizations must prioritize data privacy and security when cleansing and scrubbing sensitive data, ensuring compliance with regulations such as GDPR and HIPAA.
-
Staff Training and Change Management: Implementing data cleansing and data scrubbing processes may require training and upskilling of staff members to effectively utilize the technology and adapt to changes in workflow processes.
Best Practices for Data Cleansing and Data Scrubbing
To maximize the benefits of data cleansing and data scrubbing, organizations should follow these best practices:
-
Establish Clear Objectives: Define clear objectives and metrics for data cleansing and data scrubbing initiatives, ensuring alignment with business goals and priorities.
-
Select the Right Tools and Techniques: Choose appropriate tools and techniques for data cleansing and data scrubbing based on the specific requirements and challenges of the organization.
-
Implement Regular Monitoring and Maintenance: Continuously monitor and maintain data quality by implementing regular data cleansing and data scrubbing processes, ensuring that data remains accurate, reliable, and up-to-date.
Future Trends in Data Quality Management
Looking ahead, the future of data quality management is characterized by several emerging trends:
-
Advancements in Automation Technologies: Data cleansing and data scrubbing processes will become increasingly automated, leveraging advanced algorithms and machine learning techniques to improve efficiency and accuracy.
-
Integration with Advanced Analytics Tools: Data quality management processes will be integrated with advanced analytics tools and platforms, enabling organizations to derive actionable insights from high-quality data.
-
Adoption of Blockchain for Data Integrity: Blockchain technology will be increasingly used to ensure data integrity and traceability, providing a secure and tamper-proof way to record and verify data transactions.
Conclusion
In conclusion, while data cleansing and data scrubbing share a common goal of improving data quality, they differ in focus, scope, and methodology. Data cleansing focuses on identifying and correcting errors and inconsistencies within datasets, while data scrubbing focuses on removing irrelevant or redundant data. Both practices offer significant benefits for organizations looking to maintain data integrity and reliability, enabling informed decision-making, compliance with regulatory standards, and competitive advantage. By understanding the distinctions between data cleansing and data scrubbing and implementing best practices, organizations can maximize the value of their data assets and drive business success. As organizations continue to prioritize data quality and analytics accuracy, the integration of data cleansing and data scrubbing processes with AML software and negative data scrubbing software will be instrumental in maintaining data integrity and regulatory compliance.
What's Your Reaction?
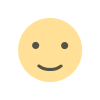
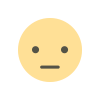
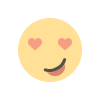

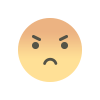

