Empowering Healthcare With Predictive Analytics
Read how predictive analytics turn your data into insights in the healthcare industry.

What is Predictive Analytics?
Predictive analytics as the name suggests is an advanced analytics technique. It predicts future events using historical data, statistical modeling, data mining methods, and machine learning. It entails looking for trends in historical data to predict future developments, spot dangers, and find openings. As it frequently involves handling and analyzing massive volumes of data from multiple sources, this discipline is strongly related to big data and data science
Importance of Predictive Analytics in the healthcare industry
Clinical treatment and operational efficiency depend on predictive analytics solutions being applied in healthcare. When healthcare providers can accurately identify health risks, they can provide patients with more reasonably priced treatment plans that extend their lifetime. Recent research shows that predictive analytics using social and environmental patient data helps one get better risk estimations for cardiovascular disease. These findings help clinicians stop at-risk patients from having worsening symptoms that can call for hospitalization.
Key components of Predictive analytics in healthcare
Predictive analytics in healthcare includes the following to gain predictive insights:
- Patient Data: For making decisions, it must have both historical and current data
- Data Management: Data must be collected and cleaned for accurate predictions.
- Analytical Models: These are the frameworks that are required to process data to make predictions.
- Technology Infrastructure: Strong systems to handle large volumes of data
- Compliances: Adherence to laws and regulations for example related to patient data privacy must be adhered
- Regular Testing & updation: Models must be regularly updated as predictive analytics is an ongoing process.
Challenges of using predictive analytics in healthcare
Predictive analytics has immense promise to transform healthcare, but various issues must be resolved even if it can do so.
- Data Quality: Predictive models depend on vast, varied datasets to guarantee accuracy and generalizability, therefore reflecting data quality and availability. However, healthcare data sometimes suffers from bias, incompleteness, and inconsistencies that might compromise predictive models' efficacy.
- Privacy and Security Issues: Predictive analytics' usage of patient data begs serious privacy issues. First and most important is ensuring the confidentiality of private health data and following HIPAA rules.
- Resistance to Change: Healthcare workers could be reluctant to embrace new technologies, particularly if they need fresh training or challenge accepted procedures.
- Ethical Issues: Using predictive analytics raises ethical issues like possible data biases that can result in unequal treatment of patients.
- Regulatory Compliances: Following the several rules controlling healthcare data and its application in predictive analytics presents a difficult and continuous task.
- Outcome Analysis: After forecasts are generated, it is crucial to examine results to evaluate the accuracy of the models and hone them for next use—a difficult process.
- Complexity: Predictive analytics can be resource-intensive and calls for advanced technology infrastructure and knowledgeable staff to manage and understand the data.
Future of predictive analytics
With developments that would improve predictive analytics' accuracy, speed, and scope, its future seems to be an interesting frontier. These important trends and innovations are likely to propel predictive analytics' development:
- Ethical use: Predictive analytics's increasing potency will lead to more attention to ethical issues and appropriate data use to avoid prejudices and guarantee justice.
- New Technologies like AI& ML Integration: Using artificial intelligence (AI) and machine learning (ML) models is becoming more common since they provide better accuracy and can be implemented by experts with different degrees of knowledge.
- Increased automation: Predictive analytics automation will speed up and simplify the process, enabling companies to make decisions faster.
- Market Growth: With estimates showing a reach of US$35.45 billion by 2027, expanding at a compound annual growth rate (CAGR) of 21.9%, the market for predictive analytics is predicted to develop dramatically.
- Advanced Algorithms: Gradient boosting, which aggregates several smaller models to produce a stronger predictive model, is becoming popular and most certainly will get more advanced.
Conclusion
Ultimately, predictive analytics in healthcare is a fast-developing discipline poised to change the medical environment. Leveraging data, statistical models, and machine learning solutions helps one to forecast patient outcomes, customize treatment approaches, and enhance general healthcare delivery using these tools. As technology develops, predicting instruments become more precise and easily available, so suggesting a bright future.
Still, the road presents certain difficulties. Problems including data protection, healthcare practice integration, and guaranteeing fair treatment need attention. The emphasis will be on developing predictive analytics solutions going forward that not only are technologically advanced but also ethically sound and advantageous to every healthcare stakeholder.
A healthcare system that can predict requirements, stop negative outcomes, and give patients prompt, customized treatment is the ultimate aim. Predictive analytics may very well be the lighthouse pointing us toward this goal of a wiser, more proactive healthcare future through ongoing innovation and responsible AI development.
What's Your Reaction?
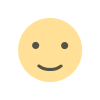
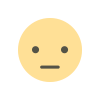
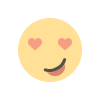

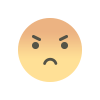

